The impact of big data on storage, in practical terms
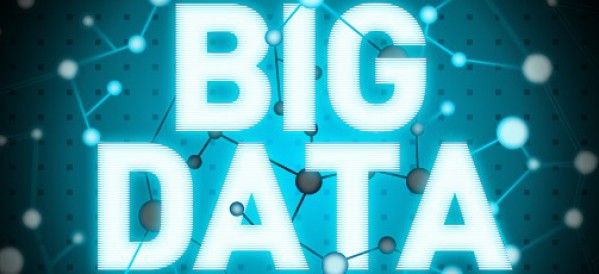
Its implementation “may” require that you choose to federalize the data, use memory storage, include scalable analytical processing, etc.
The big data, such as cloud does not refer to a particular technology, or a set of technologies. The Big Data defines a class of problem management information that is difficult or even impossible to solve efficiently using conventional tools and data management techniques.
The Big Data is commonly characterized by the five Vs: volume, speed, range, accuracy and value. The first three Vs are certainly common, while the last two are becoming increasingly evident in the vernacular of Big Data. There is a good chance that the following points characterize the kinds of challenges that the five Vs may be causing in your organization.
VOLUME of the information that you are accumulating burden the people, processes and technical capabilities of your group Enterprise Information Management. Chances are you’ll need to find a set of tools and techniques distintualmente (I know, that word does not exist, but should) different to solve this problem.
SPEED increasing with which people expect you to analyze and process the data is already exceeding the skills of its staff and its infrastructure.
You wish it the scope of the analysis was limited to a small set of data warehouses, but the reality suggests that this scope includes a VARIETY of data types, including call recordings, text documents, sensor logs, tweets, tanned, security videos of shops etc.
Some parts of your organization have doubts about the VERACITY of facial recognition in data security videos. Store video files is easy; accurately identify a face in one of a video frame is anything but easy.
Phrases like “the data is our greatest source of untapped VALUE” are heard every day in your organization. The people who produce these phrases when pressed to quantify or explain the data value, are remarkably silent.
Still reading? Good.
I would not be surprised to discover that the MIS, the Business Intelligence and Business Analytics top the attention of organizations. Would also not shocked to learn that companies still spend copious amounts of money in technology, both in solutions and in the source systems integration in BI and related systems. I would venture to say that in a very general level, the data storage architecture are as follows:
The “probably” implementations include multiple source systems (which, of course, need to be archived / protected / have made back up), staging areas for a variety of transformations and quality improvement operations, one to serve as an active EDW container structured information, and many data marts to be used for specific measurement tasks. Of course, all this complexity is perfectly automated and fully documented. There are still IT operations that are not fully automated and documented?
Would it be great if organizations NOT have a problem of Big Data. The architecture described above works well when companies have data sets of homogeneous sources and relatively small. However, say the business is trying to enter a new value in the organization that “moves” to the type of information that IT will have to go to “drive”. A new mobile application that is perhaps deployed to millions of customers, who need to have impacts in REAL TIME in the organization’s business. Perhaps the general trend of social conversations need to be analyzed alongside or against the trend of thousands of recorded call center interactions. Well, in this case, the IT will be more concerns in the design. Consider:
1 – Volume: Each additional repository data from the source system to the data mart, this architecture may need to store petabytes of data instead of terabytes.
It is impracticable to maintain operational and offsite backups to sets of multiple and massive data.
Making Storage data stream to the processing machine (for example, RDBMS) leads to an unacceptable response time to queries in the big date range.
2 – Speed: The ETL can mean a huge space between the data generating events and delivery to business customers.
The orientation “schema on write” requires initial design and analysis extensive, further delaying the derivation of value from the data.
The traditional SAN-based architecture struggles to grow fast enough to meet the demands.
A batch-oriented architecture is unable to provide insight in real time.
3 – Variety: The EDW architecture is optimized for relational data generated by business applications.
Semi-structured or unstructured data are becoming as important as structured data for analytical.
Design costs and initial analysis exacerbated by the variety in the source data sources.
Truthfulness: The lack of implicit trust in data sources requires an environment that is conductive to more agile discovery and exploration. However, the high cost of design and infrastructure inhibits agility, creating the paradox of “analysis to invest, investment to analyze.”
4 – Value: The organizations often find it difficult to find value in your data. Without careful consideration, the big data will only make the most difficult value to find.
With any hope, you will not be coming to the conclusion that the investment of your organization is a huge waste of time, money and effort (by chance you see a tsunami of Big Data coming at you …). Clearly, you are solving business problems REAL right now, and will need CONTINUE solve these problems in the future. Its current architecture will not go away, but you will need to explore and take advantage of new tools and techniques to ensure the efficient derivation of business value from this new flood of demands.
The architecture “lake date” has emerged as a response to the challenges of big data to data management architecture. Note that the date lake complements the conventional architecture of BI and analytics. Suggest a total replacement, given the substantial investment made by organizations around the world in BI, it would be madness.
A lake date (or as I call it, the lagoon of the Seven Seas) helps because: store the data in the condition they are in, on-site processing, eliminate operational backups, optimize the placement and data storage, size of simple and inexpensive way to facilitate the exploration and analysis and work on a petabyte scale.
Its implementation “may” require you to “federalize” your information source instead of center it (what you ultimately can not control or predict); include streaming or events complex processing using storage memory to manage millions of new transactions per second; include a method of packaging unstructured information and metadata storing object format for use in analytical tools; include scalable analytic processing in Hadoop clusters to create business value combined from all sources of information.
Full article: http://cio.com.br/